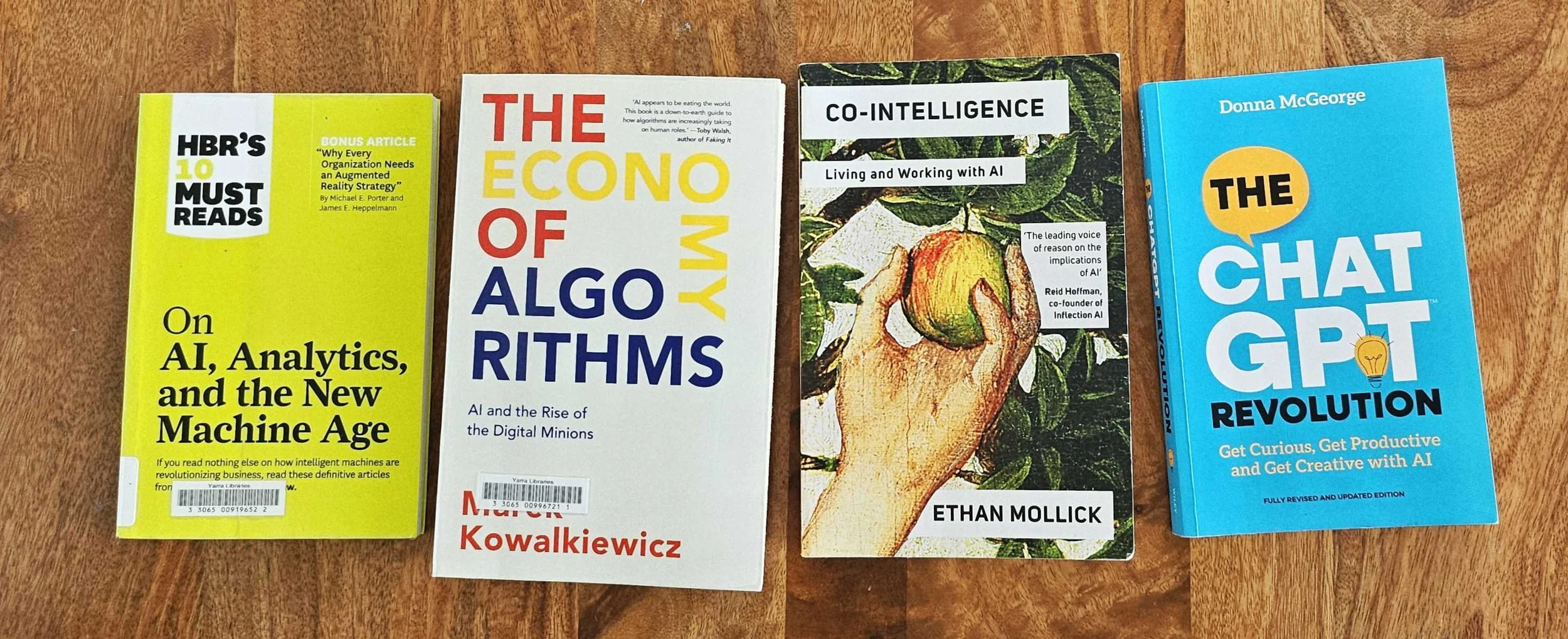
AI Book Club
I read some books about AI so you don’t have to.
Co-Intelligence: Living and Working with AI by Ethan Mollick
I devoured this book. It’s a perfect overarching overview into the ethical and practical implications of AI, with a myriad of mental models on how to integrate it into your life.
I think this book will hold up; it discusses approaches to AI in a more timeless manner that will likely mostly still make sense as AI gets more advanced.
Find it here if any of the below sounds interesting to you:
Deciding which tasks are for you and which are for AI,
His four guiding principles on approaching AI in general (more on that to come),
Three potential outcomes of AI, ranging from terrible (see: Terminator) to pretty okay,
The ethics of using AI, but in a digestible and non-scary way because somethings ethics talk gets a bit too high brow.
The ChatGPT Revolution by Donna McGeorge
This is an easy read, and my top recommendation for anyone who is worried they are being left behind in the race to become AI-competent. It absolutely does not really cover off the history, the ethics or the more esoteric discussion areas of AI, but it’s useful as hell.
Unlike some others listed here, this one will absolutely date out pretty quickly but it’s a very accessible guide to go from zero to one hundred with large language models (LLMs).
Find it here if any of the below sounds interesting to you:
The three Ps of crafting the perfect prompt (this one will be irrelevant soon as AI gets better at deciphering our terrible asks),
How to get started as someone completely terrified,
Practical examples on how to use it for work or life.
The Economy of Algorithms by Marek Kowalkiewicz
This one does exactly what it says on the tin.
It’s a great book about AI in relation to the economy and business, and a brief history of algorithms more broadly with a lot of Australian references.
I’d recommend this to senior decision makers in business who would likely sign off on AI use but might not use it heavily in their day-to-day. Great for anyone who is also a bit wary of AI and how it actually works for commercial activities, but written in a very non-judgemental way.
Things that stuck with me from this book…
Some examples of algorithms going wild (especially AI to AI with little human oversight)
Marek’s nine rules for the age of algorithms
How to think about automation and algorithms as digital minions to do your bidding for you.
P.S. Was blessed to watch Marek speak on the most fascinating panel at Melbourne Writers Festival 2024 that very much changed my knee jerk reaction to AI.
Moral AI And How We Get There by Jana Schaich Borg, Walter Sinnott-Armstrong & Vincent Conitzer
This one is a pretty hefty read, but one of the most thought provoking books on AI that I’ve read so far. But also one that’s left me with way more questions than answers.
This beautifully written book is the brain child of a philosopher, data scientist and computer scientist, as they consider the ethics of using AI to help surgeons pick the best candidate for organ transplants. Recommend for anyone still unpacking the ethics behind AI use in the modern world, especially from the perspectives of ethics, the law, business and philosophy.
Dig in here if any of the below resonates:
What is AI? (Surprisingly, there are so many different ideas and thoughts here.)
How does AI interact with the basic moral values of safety (e.g. self driving cars, medical diagnoses), equality (e.g. not adopting gender or race bias that’s present in training data), privacy (e.g. can sensitive training data accidentally get leaked), freedom (e.g. can a government track and stop people from moving around, but also AI to help the blind navigate the world freely), transparency (e.g. does the AI have to explain how it came up with something), and deception (e.g. deepfakes or AI hallucinations).
Can AI respect privacy? (I learnt some very surprising and scary hacks around reverse engineering private information from data.)
Who is responsible when things go wrong, such as a self-driving car running someone over? What are the differences between moral obligations, legal responsibilities
How do we impart human morality onto AI?
You’ll notice that these are all questions, because frankly this book just raised more questions than answers in my head.
The book ends discussing how to “bridge the moral AI principle-to-practice gap,” or, in simpler terms, getting the world to bring AI ethics into what actually happens. They have
Scale moral AI technical tools, so they’re easy check points baked into the AI development and deployment at scale.
Disseminate practices that empower moral AI practices, which is easier said than done when businesses are generally designed around stakeholder/shareholder management and profits. Other issues mentioned include how agile-lean methodologies might not easily fit AI ethics into their workflow, and figuring out who is responsible for AI ethics.
Provide career-long training opportunities in moral systems thinking, similar to how doctors and lawyers need to re-train at regular intervals to keep up to speed with changing industries.
Engage civic participation throughout the life cycle, although in an earlier chapter they discuss the balance of bringing in laypeople but also recognising that those with less experience or knowledge may have skewed context.
Deploy agile public policy, because smaller businesses without the budget to payroll an ethics or AI officer would find government best practice guidelines and policies helpful to not just survive but also thrive. Because strong AI use is good for economies.
This book covers very similar subject matter to my last AI read (Moral AI And How We Get There) but in a vastly different way. Both discuss the ethics of AI in terms of privacy, transparency, fairness and justice, but refreshingly with very different views on the all.
While Schaich Borg and friends seem to err on the side of pushing for transparency with algorithms (to be able to explain how an AI has come to a decision), Walsh disagrees. His stance is that we should be building trust between users and the AI at large, instead of a blanket rule of transparency. Transparency across the board has issues, like IP and to prevent bad actors for manipulating AI for nefarious purposes.
Another stark contrast was whether we approach what is right through the lens of fairness or equality, which at face value may seem like synonyms but in reality can have very different outcomes. A very reductive definition (sorry) for equality is everything being roughly the same for everyone to level the playing field, but fairness seems to be more nuanced, and takes other factors into consideration. As Walsh so beautifully illustrates with this example: in 2012 the Eu mandated that insurance companies charge all genders the same for equality. Sounds fair, yeah? What happened in reality was that women ended up paying higher premiums to subsidise men’s poor driving, because men are far more likely to get into accidents than women. The same goes for AI, sometimes a one-size-fits-all approach might not be right.
I enjoyed how Walsh laid out his lessons for each chapter, and there were far too many to list, but here were some of the more salient for me:
Be wary of machine-learning systems where we lack the ground truth and make predictions based on proxy for this.
Do not confuse correlation with causation, because AI systems with this may further perpetuate society’s biases.
Fairness means many different things to do people/cultures/communities/industries, and often they can be at odds with each other. This may require trade-offs.
Building trust in AI is key. Do this through explainability, auditability (think a little black box for robots), robustness (not breaking with little changes), correctness, fairness, respect for privacy and transparency (which Walsh again reminds us is overrated).
We judge AI so much harder than humans, but Walsh notes that this is not a double standard. He writes: “We should hold humans to a higher standard than humans. And this is for two important reasons. First, we should hold them to higher standards because machines, unlike humans are not and likely can never be accountable. We can put up with a lack of transparency in human decision-making because, when things go wrong, we can call people to account, even punish them. And second, we should hold machines to higher standards because we can. We should aspire to improve the quality and reliability of humand decision-making too.”
Machines Behaving Badly: The Morality of AI by Toby Walsh
How AI Thinks by Nigel Toon
Still reading this one, but a delicious read so far. Nigel Toon explores the history of AI in a beautifully written narrative. Unlike many other technology writers I’ve encountered, he pays homage to the women engineers, mathematicians and scientists who have paved the way for the field. (Fun fact: this includes the 19th-century mathematician Ada Lovelace, after whom I named my pet rabbit when I was 18.)
Toon isn’t a psychologist or neurologist, but you would be forgiven for thinking so with how brilliantly and effortlessly he explains the human brain as the inspiration for many AI frameworks. Topics like learning, memory, and attention are presented so clearly that you’d think he was an expert in these fields. (Although he is a scientist with patents to his name, as well as a leader and entrepreneur in the tech space, so nothing to be sneezed at.)
Some of my favourite facts so far:
Aristotle outlined two methods of answer-finding: deduction, where several statements lead to one conclusion, and induction, where observations are synthesised to form a general conclusion. Deduced answers are certain (as Sherlock Holmes would demonstrate), whereas induction is fuzzier. Answers might be right, they’re probably right, or they’re one of a few plausible options. Think: the certainty of “1+1” versus the complexity of writing an essay on intersectionality. AI researchers have applied induction to great effect, with the first “deep artificial neural network” developed in Japan in the late 1970s. Teams in Canada and Switzerland further advanced this work over the following decade.
The US-USSR race to the moon was a major catalyst for the technology that allows me to type this on a flat laptop while sipping a latte in a cafe. Planar microchips were initially prohibitively expensive, costing over $1K each. However, US President Kennedy’s push for the Apollo Program created a significant demand, eventually driving costs down.
Ada Lovelace (my pet bunny’s namesake, if you recall) first conceptualised the use of a high-level programming language for practical purposes. Remarkably, this was before her death in 1852—nearly a century before the first electronic computer.
Highly recommend this one for anyone who loves a good psychology read, and loves to understand the context and history behind things.